
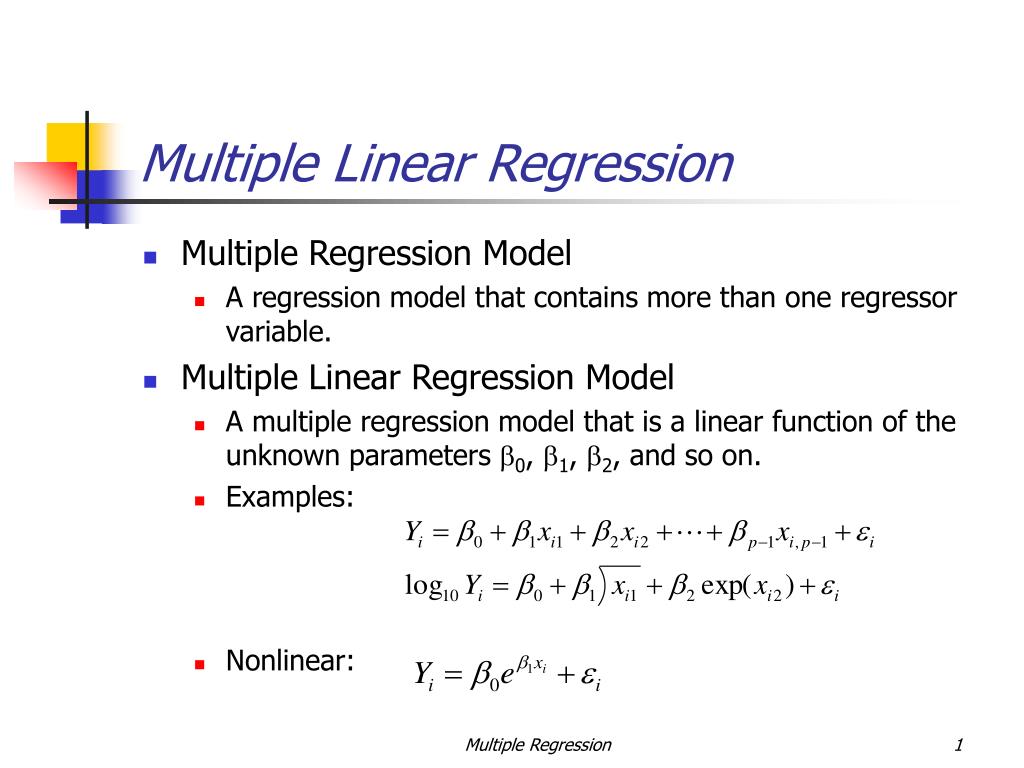
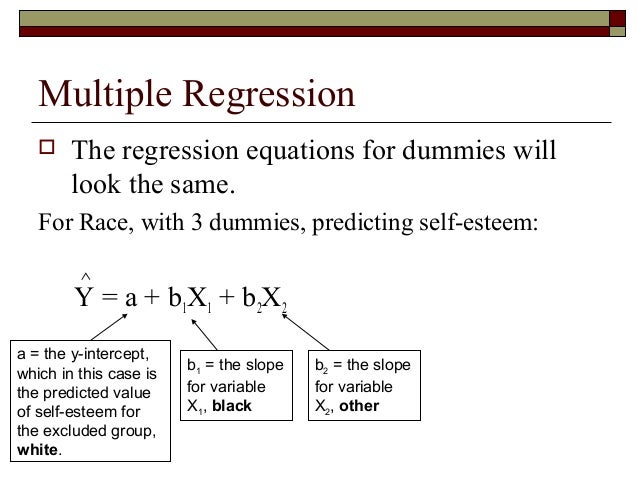
#Multiple linear regression equation example how to
General accounts on how to treat such dependent categorical variables in the context of exploratory factor analysis, and hence to SEM more generally, are given by Flora and Curran (2004), Jöreskog and Moustaki (2001), and Moustaki (2001). Even three-category data treated continuously can perform well enough ( Coenders et al., 1997), but we do not recommend it as routine practice. The remaining case is when a dependent categorical variable is either binary or with three categories. Second, if a dependent categorical variable is ordered and has at least 4 or 5 categories as in a typical Likert scale, treating it as a continuous variable will create few serious problems (e.g., Bentler and Chou, 1987). It is common that independent variables are categorical in multiple regression, and SEM can handle such variables by dummy coding as is done in multiple regression.
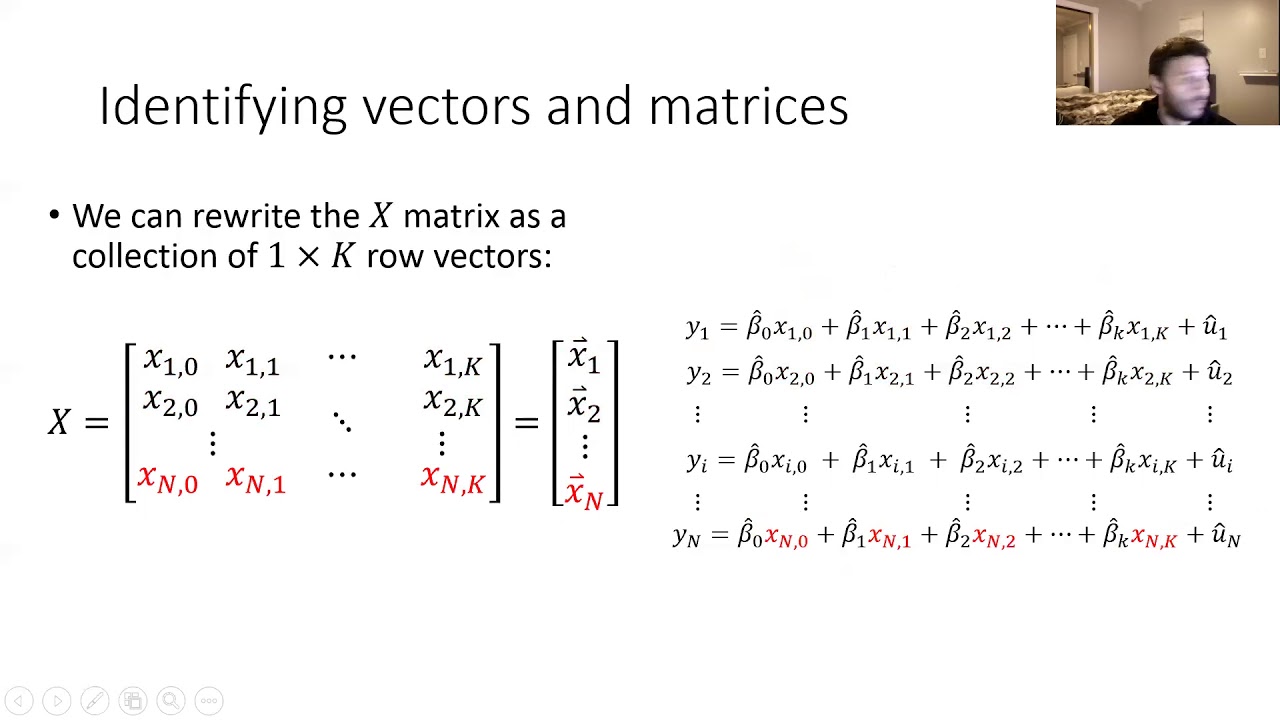
First of all, note that no special methods are needed if the categorical variables are independent variables. Categorical variables are frequently used in medical and epidemiological research. This may not always hold true in practice. So far, we have assumed that the observed variables are continuous. It also suggests that, if they had estimated the equation with the school resources variables first and then added the student background variables, they again would have found little change in R 2, suggesting that it is student backgrounds, not school resources, that “show very little relation to achievement.” More generally, this example shows why we should not add variables in stages but, instead, estimate a single equation using all the explanatory variables. Multicollinearity explains why the addition of the school resources variables had little effect on R 2. The student background variables were positively correlated with the school resources variables, and this multicollinearity problem undermines the Coleman Report's conclusions. Because the inclusion of the resources variables had little effect on R 2, they concluded that school resources “show very little relation to achievement.” The problem with this two-step procedure is that school resources in the 1950s and early 1960s depended heavily on the local tax base and parental interest in a high-quality education for their children. They then reestimated the equation using the student background variables and the school resources variables. The researchers first estimated their equation using only the student background variables. Some explanatory variables measured student background (including family income, occupation, and education) and others measured school resources (including annual budgets, number of books in the school library, and science facilities). One of the more provocative findings involved a multiple regression equation that was used to explain student performance. In response to the 1964 Civil Rights Act, the US government sponsored the 1966 Coleman Report, which was an ambitious effort to estimate the degree to which academic achievement is affected by student backgrounds and school resources. Gary Smith, in Essential Statistics, Regression, and Econometrics (Second Edition), 2015 The Coleman Report All of the techniques you learned earlier in this chapter can be used: predictions, residuals, R 2 and S e as measures of quality of the regression, testing of the coefficients, and so forth. More precisely, you have a linear relationship between Y and the pair of variables ( X, X 2) you are using to explain the nonlinear relationship between Y and X.Īt this point, you may simply compute the multiple regression of Y on the two variables X and X 2 (so that the number of variables rises to k = 2 while the number of cases, n, is unchanged). 26 This is still considered a linear relationship because the individual terms are added together. With these variables, the usual multiple regression equation, Y = a + b 1 X 1 + b 2 X 2, becomes the quadratic polynomial Y = a + b 1 X + b 2 X 2. Let us consider just the case of X with X 2. You are using polynomial regression when you predict Y using a single X variable together with some of its powers ( X 2, X 3, etc.). You now have a multivariate data set with three variables: Y, X, and X 2.
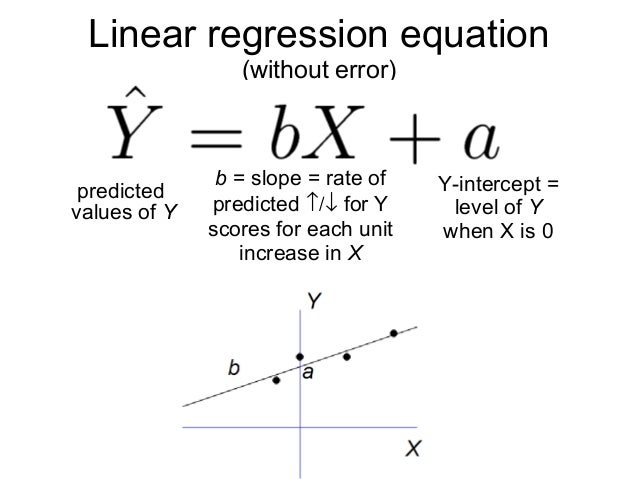
The simplest choice is to introduce X 2, the square of the original X variable. If the scatterplot of Y against X shows a curved relationship, you may be able to use multiple regression by first introducing a new X variable that is also curved with respect to X. Wagner, in Practical Business Statistics (Eighth Edition), 2022 Fitting a Curve With Polynomial RegressionĬonsider a nonlinear bivariate relationship.
